Bibcode
Mong, Y. -L.; Ackley, K.; Galloway, D. K.; Killestein, T.; Lyman, J.; Steeghs, D.; Dhillon, V.; O'Brien, P. T.; Ramsay, G.; Poshyachinda, S.; Kotak, R.; Nuttall, L.; Pallé, E.; Pollacco, D.; Thrane, E.; Dyer, M. J.; Ulaczyk, K.; Cutter, R.; McCormac, J.; Chote, P.; Levan, A. J.; Marsh, T.; Stanway, E.; Gompertz, B.; Wiersema, K.; Chrimes, A.; Obradovic, A.; Mullaney, J.; Daw, E.; Littlefair, S.; Maund, J.; Makrygianni, L.; Burhanudin, U.; Starling, R. L. C.; Eyles-Ferris, R. A. J.; Tooke, S.; Duffy, C.; Aukkaravittayapun, S.; Sawangwit, U.; Awiphan, S.; Mkrtichian, D.; Irawati, P.; Mattila, S.; Heikkilä, T.; Breton, R.; Kennedy, M.; Mata Sánchez, D.; Rol, E.
Bibliographical reference
Monthly Notices of the Royal Astronomical Society
Advertised on:
10
2020
Citations
13
Refereed citations
10
Description
The amount of observational data produced by time-domain astronomy is exponentially increasing. Human inspection alone is not an effective way to identify genuine transients from the data. An automatic real-bogus classifier is needed and machine learning techniques are commonly used to achieve this goal. Building a training set with a sufficiently large number of verified transients is challenging, due to the requirement of human verification. We present an approach for creating a training set by using all detections in the science images to be the sample of real detections and all detections in the difference images, which are generated by the process of difference imaging to detect transients, to be the samples of bogus detections. This strategy effectively minimizes the labour involved in the data labelling for supervised machine learning methods. We demonstrate the utility of the training set by using it to train several classifiers utilizing as the feature representation the normalized pixel values in 21 × 21 pixel stamps centred at the detection position, observed with the Gravitational-wave Optical Transient Observer (GOTO) prototype. The real-bogus classifier trained with this strategy can provide up to $95{{\ \rm per\ cent}}$ prediction accuracy on the real detections at a false alarm rate of $1{{\ \rm per\ cent}}$ .
Related projects
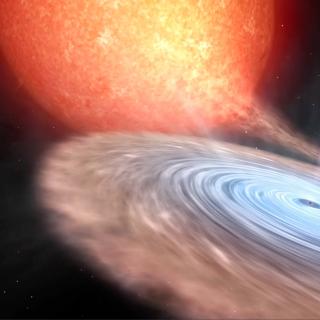
Black holes, neutron stars, white dwarfs and their local environment
Accreting black-holes and neutron stars in X-ray binaries provide an ideal laboratory for exploring the physics of compact objects, yielding not only confirmation of the existence of stellar mass black holes via dynamical mass measurements, but also the best opportunity for probing high-gravity environments and the physics of accretion; the most
Montserrat
Armas Padilla
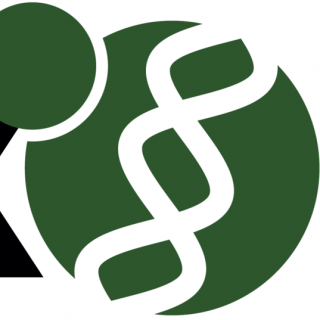
Exoplanets and Astrobiology
The search for life in the universe has been driven by recent discoveries of planets around other stars (known as exoplanets), becoming one of the most active fields in modern astrophysics. The growing number of new exoplanets discovered in recent years and the recent advance on the study of their atmospheres are not only providing new valuable
Enric
Pallé Bago